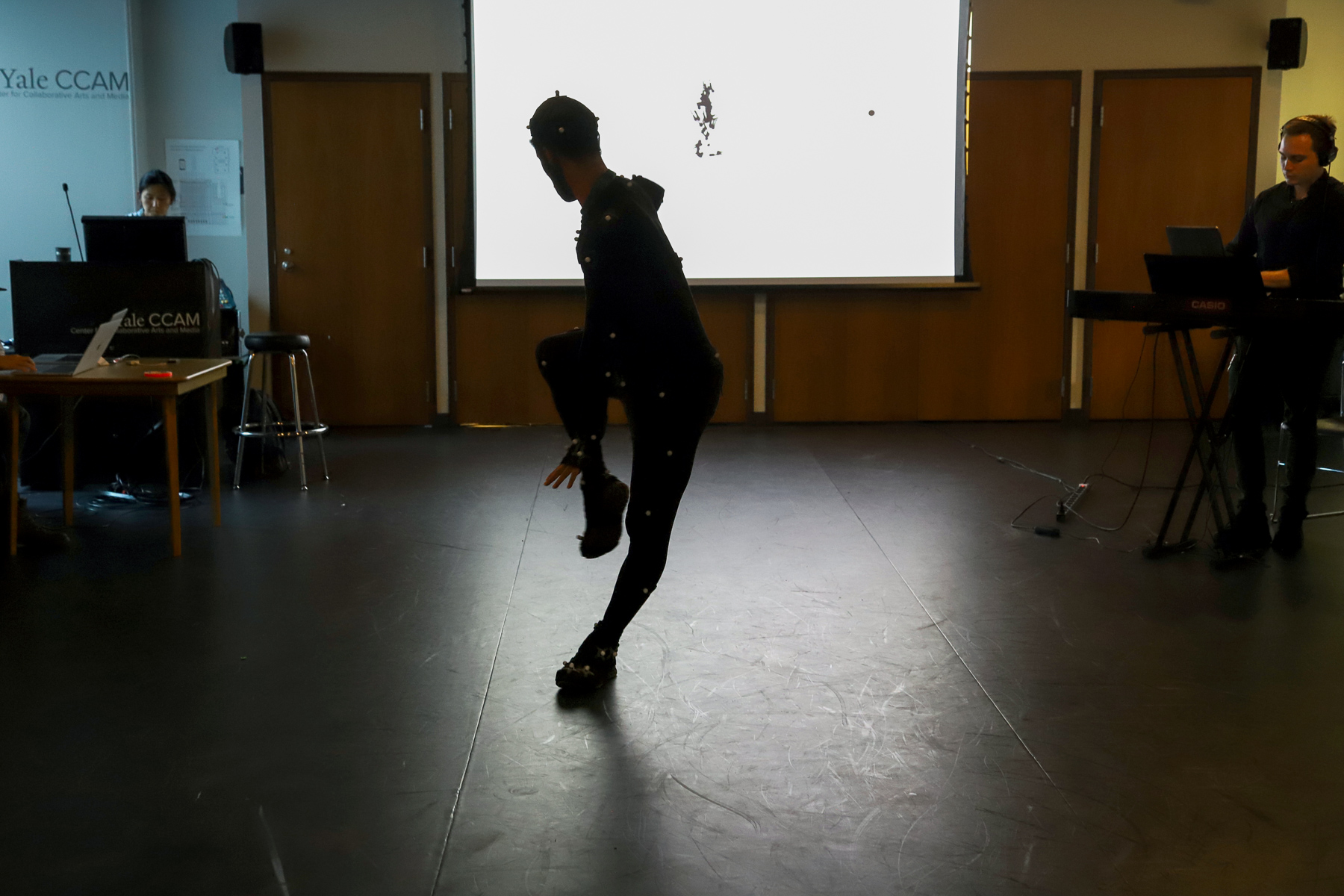
Dance with Machine, from the IEEE Games Entertainment and Media Conference at CCAM, 2019.
All Connected:
The Dance with Machine Performance at IEEE GEM 2019
Tianshu Zhao
The Dance with Machine Performance at IEEE GEM 2019
Tianshu Zhao
My hands were a little stiff during the performance. I had ten fingers to interact with three computers, five software programs, and a ten-channel mixer, which—along with thousands of circuits in cameras, speakers, and projectors—became the conduit between Raymond’s movement and my audiovisual experience.
Raymond Pinto is a highly trained Broadway dancer and Julliard alumnus, and he had warned me this project would not be easy at all. We were presenting the piece at the Institute of Electrical and Electronics Engineers (IEEE) Games, Entertainment, and Media (GEM) conference. This year it was held and produced at CCAM with an extensive and inspiring roster. I was the specialized technology facilitator at CCAM, and I worked collaboratively with the Yale community, with both students and faculty members, on their interdisciplinary arts and technology projects, which included motion capture, augmented reality, virtual reality, filmmaking, photography, graphic design, printing, sound design, covering everything from ideation to implementation.
Raymond and I met last May at CCAM. He asked whether I would be interested in collaborating on a performance involving machine learning and choreography for the conference. At that time, I was involved in another machine learning research project on the relationship between human language and movement, so naturally, I was intrigued. Raymond had been exploring the idea of digitizing his movement using technological means substantially to “go past the boundaries of his body” for some time. Motion capture was one of his most recent explorations.
I have always been fascinated by how audiovisual experience designers can create interactive space and immerse viewers or listeners into a digitized world. The idea came to my mind the first time I saw the 22-camera Vicon Motion Capture system in the Leeds Studio at CCAM. I wanted to connect human input and some forms of digital output to create something visually and auditorily interesting. Motion capture is the technology used widely in animations, video games, kinetic studies, and computer science to accurately record the movement of humans and/or objects. Here at CCAM, the interdisciplinary hub at Yale, the Leeds Studio was the perfect playground to conduct experimentations on visualization, music composition, and beyond with humanoid movement as data sources. And the Beyond Imitation research project incubated by Blended Reality program, an applied arts research project that explores the possibilities of mixed reality technologies partnered by Yale and HP and hosted at CCAM since 2016, provided an even better opportunity for me to dive deeper into the ideas.
The research for Beyond Imitation was originally conducted by Mariel Pettee (PhD Candidate, Department of Physics, Yale University), Chase Shimmin (Postdoc, Department of Physics, Yale University), and Douglas Duhaime (Digital Humanities Lab, Yale University) with two brilliant dancers Ilya Vidrin (Harvard Dance Center, Harvard University) and Pinto. It uses recurrent neural networks and autoencoder architectures to investigate questions about how humanoid movement can be translated and transformed by algorithms, and how we can utilize and interact with machine learning output. The goal of this research is to create open-source tools for the research and the practice of experimental dances; the goal of the performance was to demonstrate the research result in front of other scholars and, more importantly, to test whether the tool can generate effective human-machine collaboration.
The main idea was rather simple: We wanted Raymond and the neural network to learn from each other in real-time and in the same place, and to see what could be generated out of this mutual learning process. Raymond and I began this project without knowing whether we would be able to achieve it. Shimmin and Duhaime came on board later in the process and brought a lot of the technical details from the original research on the table.
In the beginning, Raymond challenged himself and improvised for hours and hours in the afternoon rehearsals. I did some visual experiments by shifting perspectives and rendering different formats of captured motion data to interact with him. Gradually we added dance phrases generated by the neural network, which was when Raymond started to feel awkward. The algorithm could swallow whatever it was fed, but for human Raymond, it was difficult to communicate with or embody such algorithmic output. Visually, we were hoping to see a series of smooth communications between the human dancer and the generated dancer.
The goal was only halfway achieved: Neither Raymond nor the neural network could learn fast enough to have a meaningful output on-site. In other words, they needed time mentally and physically to process what they saw. However, for us designers and researchers, this was the fun part. Chase had showed us how he trained the neural network and how it learned little by little from Raymond’s movement data—“Look how the neural network became more and more confident in imitating the turn and the slide!” It was fantastic to hear him using the anthropomorphized word “confidence” to describe an algorithm. But, when will we be able to feel comfortable to recognize machine intelligence or feel confident in learning from it? As a result, on the day of the presentation, we let the neural network only decode (understand without putting it into practice) Raymond’s captured movement, but didn’t ask it to encode its own version.
The sound was another layer in the performance. As another dimension of the experiment, Raymond’s movement was translated into a sine wave sound with the volume controlled by his center of gravity and the frequency by the distance of his hands. Some parts of the sound layer were hidden, because the last thing we wanted to do was to confuse people with too many things to process. The beginning and the ending music piece was composed by me using the Watson Beat algorithm based upon Le Tombeau de Couperin by Maurice Ravel, performed by Maxwell Foster (Australian classical pianist, Juilliard and Yale alumnus). I intended to use the music composition process to parallel the research which was focused on choreography. It was almost a game we played with the audience. Were they aware that the music was composed by me and a piece of code? Would they feel or respond differently if they knew?
By the time the show ended, I forgot all about my stiff fingers and began to experience the performance with the audience, and observe them secretly at the same time. I couldn’t take my eyes away from the two-dimensional latent space (the decoded movement information), and in my head, tried every way to connect it to the 159-dimensional (53 markers times three translation coordinates) movement data. The audience was probably overwhelmed because the performance was loaded with information—the moving body, the captured movement data in different formats of 3D animations, the 2D latent space, the generated sine wave sound, not to mention the midi keyboard mix by a classical pianist.
I appreciated the fact that the performance was so intriguing that, with or without the pre-knowledge of the research, we were all curious about what was happening and what would happen during the process. Professor Kathleen Ruiz from the Department of the Arts at Rensselaer Polytechnic Institute asked me about where the music came from and praised its integrity, without knowing that it was collaboratively composed by me and the algorithm. It motivated me to keep doing what I have been doing: creating with machines. Going back to my fascination about the interactivity between human and machine intelligence in the realm of design and arts, it is about how we can be connected to the world and the universe beyond our natural senses and our natural capabilities.
All of our attempts raised similar questions: how do we, as humanoid, interact with machines? What does creativity mean? What are our relationships with machines? Will such relationships change our view about who we are and where we are going? Will there be a time when Raymond and the neural network can choreograph a piece of dance on-site in real-time and interact with each other seamlessly? I would say yes, if they take time to get adequately familiar with each other and they are mentally and physically prepared on the performance day.
The goal is not about getting a hundred percent accurate imitation. It’s a duet. We, machine and us, can focus on what we are good at.